Manufacturing Prognostics for SMEs (published in FMPro 12/2022)
Intelligent production machines are no longer just a pipe dream. Customized artificial intelligence (AI) solutions are increasingly encountered in today's production lines. In the past two years, we have seen an increasing number of young manufacturing prognostics services of equipment and machine manufacturers. Most recently, Trumpf integrated a machine monitoring service into its Oseon software. EMAG's Edna Health Check includes a similar value proposition. An analysis of the product portfolios of Swiss machinery companies shows: In Switzerland, about 13% of mechanical engineering companies market data-driven solutions at an advanced level. Few fall into the class of small and medium-sized enterprises (SMEs).
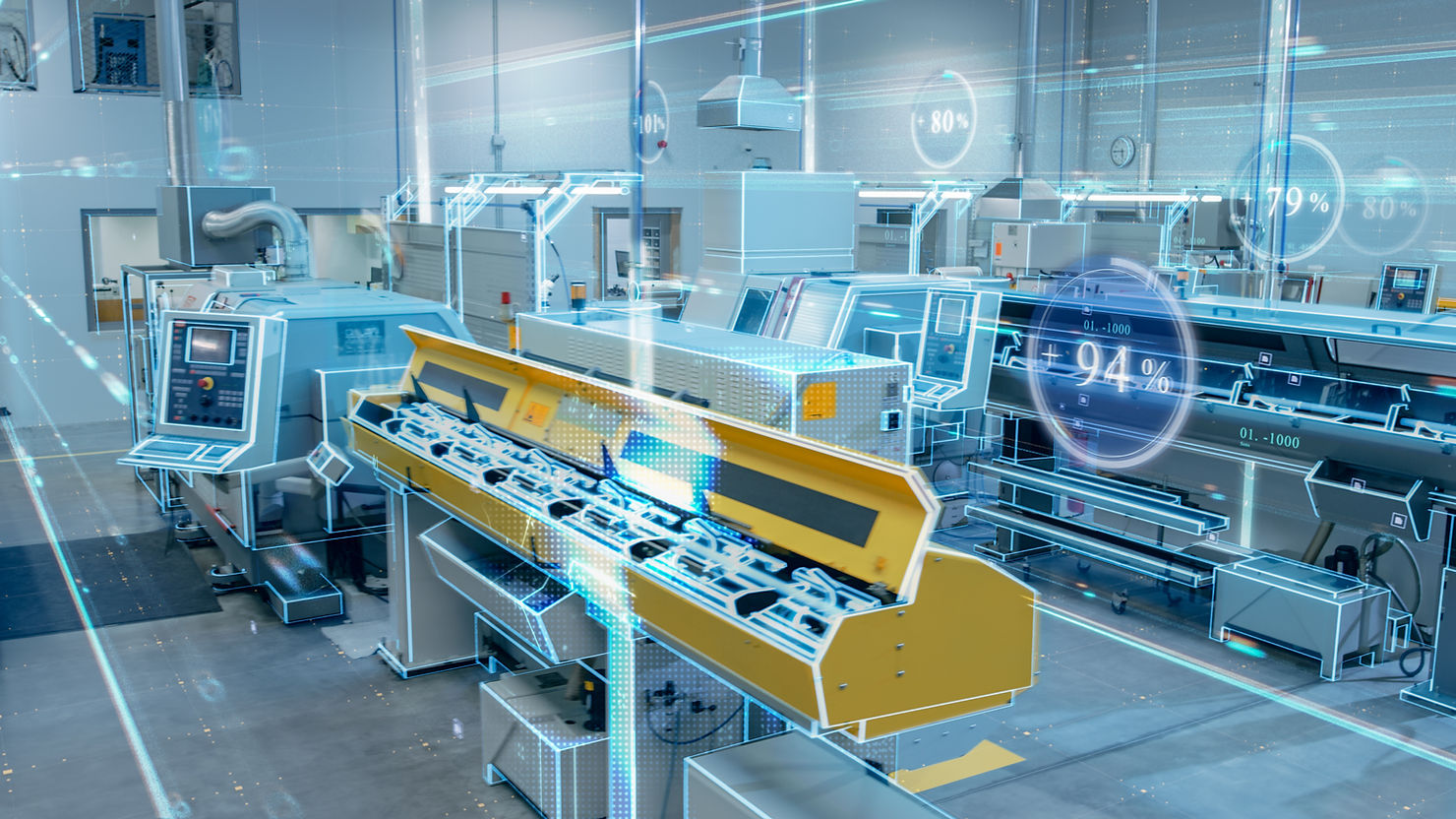
Artificial intelligence - only an issue for the industry's largest?
"According to Julius Kirschbaum, this statement is simply wrong: "Medium-sized companies can't use AI. The industrial engineer knows what he is talking about. As an innovation researcher at the Chair of Information Systems in Nuremberg, he deals with the digital transformation processes of manufacturing companies. In a consortium of industry and research, he is supporting the development and introduction of IIP-Ecosphere, a data platform that aims to set new standards in terms of open innovation in digitization.
When it comes to the term "SME" - almost 60% of the value added contribution of Swiss industry falls into this category - it is already necessary to differentiate, he says. There is no such thing as the SME. The regionally established automation company, the highly specialized component manufacturer whose product can be found as a little-known but indispensable component in many manufacturing systems, and the globally active microtechnology manufacturer all fall into this class - to describe them as diverse would be an understatement.
The digitization advantage of certain industries is obvious: the starting position of an electronics manufacturer is by nature quite different from that of a steel mill. However, this should by no means be generalized. Pioneers can be identified in all industries, says Mr. Kirschbaum. He looks for patterns and success factors. What can be seen in many of the early AI entrants is a pronounced self-image of the company as a digital organization. The strategic decision to develop AI-supported processes and services is obvious in these organizations and fuels the long-term company-wide transformation process.
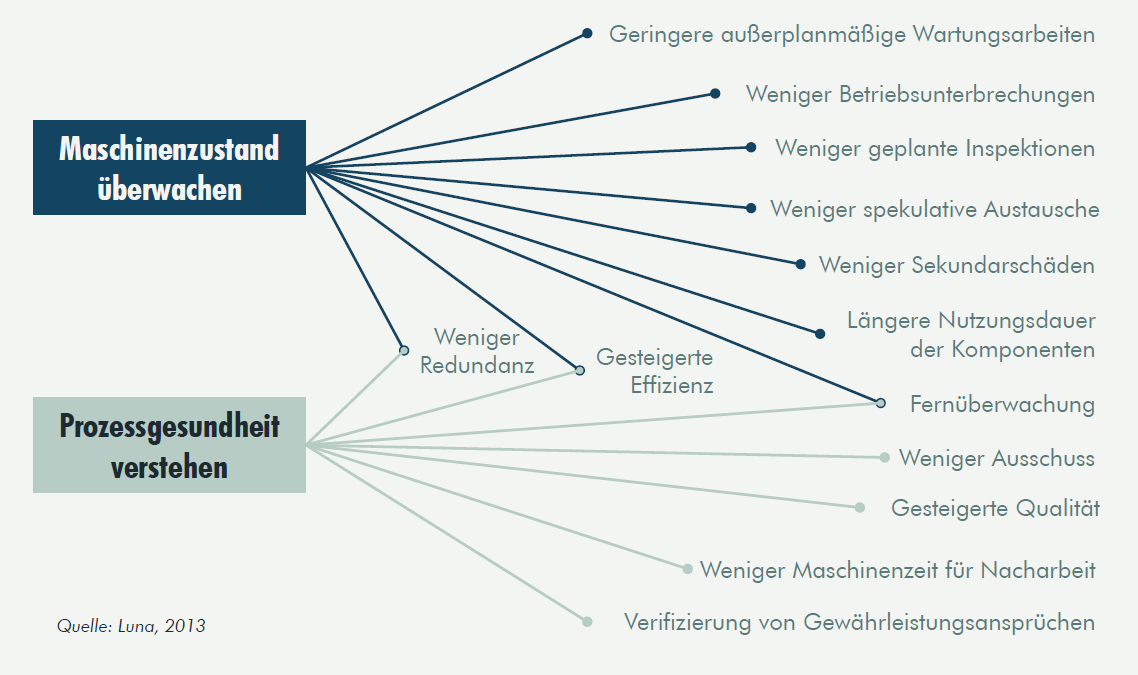
Extracting more value from the data of one's own manufacturing processes - hardly any top-level manager will not have at least thought about this wish in recent years. The sensors of modern manufacturing plants record hundreds of data points every millisecond. Hidden in these data is highly up-to-date information about the health of the company's own equipment and the quality of its own processes. If sufficient data is available, modern AI methods are able to make this hidden information usable. Thanks to such manufacturing prognostics solutions, machine failures can be predicted, component lifetimes can be extended thanks to predictive maintenance strategies (predictive maintenance), or quality deviations in process data can be detected and localized at an early stage (predictive quality). Not surprisingly, some industry thought leaders are quick to proclaim data as the new oil. It's just that crude oil is fairly limited in its usefulness. So it is with this new resource. Without the appropriate data pipelines, processing and storage, the proprietary AI solution remains wishful thinking.
Early adopters first invested in their data architecture
To harness their data capital, early adopters first invested in developing their own data architecture. This process alone usually takes at least two years. It is accompanied by important questions: What data is stored, and where? How do you operate data-driven solutions securely in critical or sensitive manufacturing processes? Companies have learned that such questions cannot simply be outsourced to the IT department. In general, he said, this is another indicator: successful companies can already be recognized by how the IT department is integrated into the company. There are good models for assessing the digital maturity of a company, such as the Industry 4.0 Maturity Index. "However, you can often tell how digitalization is going in a company just by listening to how IT and production talk to each other," says Mr. Kirschbaum.
Barriers and success factors in SMEs
An individual team of specialists in the company is rarely enough to cover all facets of data engineering, AI development and operation. Some pragmatic SMEs may doubt the usefulness of the company's own AI program in view of the abundance of investments. Coupled with a host of unanswered legal questions surrounding AI deployment and an unclear business model, AI development is still on the back burner at many companies.
"We talk to hardly any company where you can't find somewhere the dusty PowerPoint slides of a failed internal predictive maintenance project," says Till Schöpe. For several years, he developed solutions in the field of manufacturing prognostics at CSEM, a Swiss innovation incubator, and worked to help SMEs achieve industry-ready AI solutions. He is particularly saddened to see how much heart and soul has been put into the development of a working solution, only to find that the solution has never been operated beyond the proof-of-concept phase.
As founder and CTO of Alpamayo Intelligent Quality Solutions, he sees himself continuing that mission. "We want to make AI solutions more accessible to the manufacturing midmarket." Standardization of AI solutions with industrial time series data, as has been underway with image data for several years, is long overdue, he says. He is convinced: this standardization will lead to massive efficiency gains in AI development and it will allow scalable software to further reduce the currently high individual overhead in data engineering and operation of AI solutions. Proven solutions will also reduce the technological risk of AI development.
With this motivation, he manages software development at Alpamayo. He has his eye on the second movers who want to get on board with Manufacturing Prognostics now: "Many SMEs are lagging behind, but thanks to the experience of the pioneers, you can catch up all the faster. The short decision-making paths in SMEs help all the more here."
In the end, it boils down to a "make-buy-or-allie" decision, especially for SMEs, concludes Mr. Kirschbaum. While many a larger player has been able to carve out a pioneering role for itself thanks to its decision to "make," open innovation processes and the tailored offerings of startups like Alpamayo could be precisely the building blocks that will help AI achieve its moment of success in manufacturing SMEs.